Meta's 3rd version of their open language model, Llama 3 has proven to be incredibly strong as it is even competitive with some frontier models. In this blog post, we dive into some of the technical details behind Llama 3. Additionally, we provide an in-depth overview of how the open language model compares to an array of other models, while unpacking the rationale of its immense dataset size used for training and the implications this is likely to have on scaling.
20 Things You Should Know About DeepSeek
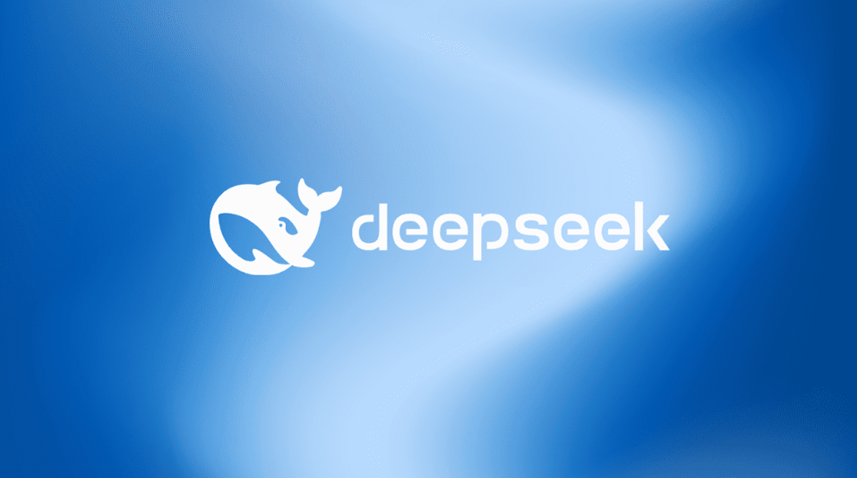
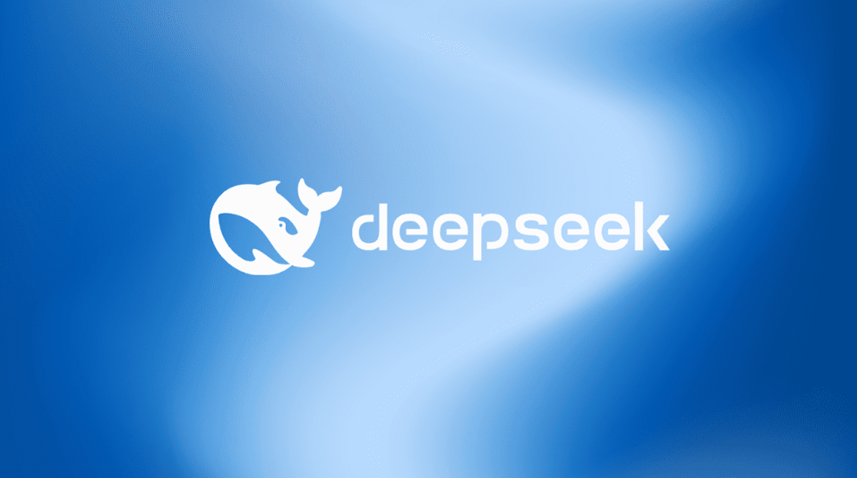
DeepSeek R1's release has triggered a seismic shift in the AI landscape. Within just 15 days, their AI assistant topped app stores across 140 markets, surpassing 30 million daily active users and breaking ChatGPT's previous adoption records. This China-born AI lab, while maintaining a low profile, has fundamentally challenged how we think about the path to AGI. Even Sam Altman acknowledged that closed-source might have been “on the wrong side of history”, with OpenAI subsequently releasing o3-mini.
Here are 20 critical insights you need to understand DeepSeek R1’s transformative impact.
1. Has DeepSeek Surpassed OpenAI?
DeepSeek has undoubtedly outperformed Meta Llama, but it still lags behind top-tier players like OpenAI, Anthropic, and Google. Take Gemini 2.0 Flash as an example—it’s cheaper than DeepSeek, highly capable, and fully multimodal. The industry has underestimated what Gemini 2.0 and other top-tier models can do simply because they haven’t been open-sourced, which is why DeepSeek’s release felt so disruptive.
DeepSeek is exciting, but it’s not yet a paradigm-shifting innovation. A more accurate way to describe it is that it open-sourced the approach that OpenAI’s o1 kept partially hidden, pushing the entire ecosystem toward broader adoption.
From a first-principles perspective, surpassing the top-tier model labs within the Transformer paradigm is incredibly difficult. There’s little room for leapfrogging on the same trajectory. What’s more exciting now is seeing who will break out and pioneer the next-generation AI architecture and paradigm.
2. How Many GPUs Does DeepSeek Have?
While Scale.ai's Alexandr Wang claimed on X that DeepSeek operates 50,000 GPUs, public data points to a more modest infrastructure: around 10,000 legacy A100 chips and potentially several thousand pre-embargo H800 accelerators. According to SemiAnalysis, DeepSeek may have bought lots of H20.
The DeepSeek team maintains strict export compliance protocols - no post-sanction chips have been procured, resulting in intentionally constrained compute capacity. Unlike its US peers, DeepSeek's resource constraints force surgical precision in infrastructure allocation, focusing on high-impact research verticals.
A big part of DeepSeek’s research is focused on finding ways to lower hardware costs, aiming to cut expenses in the largest scaling directions. Take the MLA for example. It introduces a new attention mechanism that stores all keys and values in a shared vector, allowing each token to use a smaller vector. The system only needs to store the smaller vectors, making it far more efficient. This scaling technique, especially in MoE structures, outperforms the MQA seen in Llama.
The CEO Liang Wenfeng has said it best: "In the face of hardware performance advancements, the moat created by pure algorithms is short-lived."
3. How Unique Is DeepSeek?
There are plenty of quant funds and AI labs, but DeepSeek is the logical yet surprising fusion of both. While DeepSeek operates independently, it benefits from its parent company’s first-mover advantage in AI infrastructure — securing A100 clusters for AI research as early as 2021, ahead of regional cloud providers—DeepSeek is the only non-BAT(Bytedance, Alibaba and Tencent) player with sufficient computing to compete in the foundation model race.
It perfectly solves the ‘impossible trilemma’ of a research lab: no monetization pressure, no external investors, and a CEO who is both a money-maker and an AI genius.
The quant business sustains itself, eliminating commercialization pressure (unlike OpenAI or Anthropic); it has no external investors or public listing, meaning no fundraising or investor pressure (unlike OpenAI,xAI or Anthropic); and the founder, a tech expert and businessperson, stays hands-on with model training and data annotating while driving resources (unlike Sam or Elon). This combination of these factors is incredibly rare.
That being said, the synergy of quantitative finance and scientific research has strong precedents. D.E. Shaw has made world-class contributions to molecular dynamics (Desmond Software) and high-performance scientific computing (Anton Supercomputer). Similarly, Renaissance Technologies’ founder, Jim Simons, made significant contributions to mathematics including Simons’ formula.
4. What Does DeepSeek’s “Free Strategy” Mean?
It will disrupt the ChatGPT Plus pricing and the API pricing of OpenAI and Anthropic, which is a huge benefit for all application companies. Estimates from AI coding products like Cursor and Windsurf suggest their monthly API costs are hundreds of thousands to millions of dollars. By adopting open-source R1, DeepSeek offers AI startups huge cost savings.
Cloud giants like AWS and Azure are already serving DeepSeek’s models, while startups like Perplexity and Codeium also rushing to integrate DeepSeek’s tech into their products. R1 is definitely a game-changer for AI entrepreneurs.
On the consumer side, DeepSeek’s mobile product surpassed 15 million DAU in just 18 days(and now 30 million) after its launch, whereas ChatGPT took 244 days to reach the same milestone—making DeepSeek 13 times faster than ChatGPT.
The implication is that AI technology advances so rapidly that few products can build lasting moats. Whether it’s the early reputation of Chatbot/Sonnet, developer tools like Cursor/Windsurf, early adopters have zero loyalty when something better comes along. Right now, AI product defensibility is weak across the board.
However, DeepSeek has very limited compute resources, and they clearly weren’t prepared to handle this surge in traffic. Trying to balance user inference, research experiments, and the pursuit of AGI with such constraints is nearly impossible. As a result, DeepSeek may struggle to sustain its rapid user growth, while players with more compute—like OpenAI, Anthropic, and even Meta—could ultimately benefit by improving their models and lowering costs.
5. Why Is DeepSeek’s Cost So Low? Does It Have a Gross Margin?
Based on public information and interviews, we are confident that DeepSeek is not losing money to subsidize users. DeepSeek likely operates with a positive gross margin for its AP. Therefore, its inference cost must be lower than the pricing we see.
R1’s price of around $2.19 per million tokens offers a massive price advantage compared to o1’s $60 per million tokens—even cheaper than GPT-4. We speculate that R1 is a distilled model of around 30B parameters, while o1 is a model of around 300B parameters. The 30x price difference comes from two cost factors: R1 is 10x smaller in model size than o1, and during the inference phase, o1 has more search attempts, contributing to a 3x difference.
In terms of cost, in the ARC-AGI experiments, o3-low costs $20 and 0.33M tokens per question, while o3-high runs up to $3400 and 57M tokens per question. This puts o3 at about $60 per million tokens—matching o1’s current pricing. If we calculate the number of tokens per CoT sample, each CoT generates approximately 55k tokens.
In response to R1’s price reduction, o3-mini has launched with an API price of $1.1/million input tokens, and $4.4/million output tokens. o3-mini is at the same cost level of R1, which implies it’s also a approximately 30B model.
6. Why Has DeepSeek Been Able to Catch Up So Quickly?
It’s simple: reasoning models require high-quality data and training. While catching up to closed-source models can be challenging, especially when dealing with long texts or multimodal data, the architecture of reasoning models like R1 remains relatively unchanged. Reasoning, therefore, is a more attainable goal for DeepSeek.
It took DeepSeek one year to catch up with GPT-4 and o1. After launching their first model in late 2023, it took just six months to catch up to GPT-4, and 12 months to catch up to OpenAI’s o1.
In fact, DeepSeek still lags behind OpenAI, Anthropic, and Google in some areas. For example, Google’s Flash 2.0 is not only more cost-efficient than DeepSeek but also fully multimodal. However, DeepSeek was the first in the industry to open-source a reasoner model and RL methods, as well as the first to fully expose CoT reasoning to users—both of which created a major buzz.
7. What’s the Cost of Post Training Data?
R1 introduces a novel paradigm to post-training by prioritizing RL from the base model over traditional SFT and RLHF. It also favors synthetic data generation over human-curated datasets, which significantly lowers the data barrier for other companies training LLMs. This represents a democratization of advanced reasoning models.
The cost of the post-training process is not detailed in the report, which includes an 800k dataset spanning multiple domains. Based on the o3 report, we estimate 55k tokens per chain-of-thought (CoT) for each data sample, which results in a total of 44 billion tokens. At an estimated cost of $2.20 per thousand tokens, this amounts to approximately $100k to generate the dataset. The synthetic data generation process also involves rejection sampling, and assuming a 10% acceptance rate—since early RL outputs are often unrefined—the cost to generate the necessary post-training data could reach up to $1 million. It’s important to note that this cost estimation is highly sensitive to the acceptance rate.
Considering that DeepSeek is conducting post-training on a relatively small scale, while OpenAI and other larger labs are running large-scale RL, the cost of post-training data could be 10 to 100 times higher than $1 million.
8. Can Distillation Surpass SOTA?
R1’s distillation approach suggests a clear pipeline for reasoning models: a large language model becomes a reasoning model through RL, and then it’s distilled into a smaller model to optimize costs. But there’s a catch—this strategy is mainly suited for followers. It’s not feasible to surpass OpenAI just by distilling large-scale data.
Distillation itself also has its hurdles. If solid data isn’t accumulated during pre-training and distillation is applied directly, the model tends to learn in the simplest way—akin to rote memorization—without truly understanding the problem-solving process or exploring creative solutions.
It’s likely that DeepSeek used some distillation data for cold-start and alignment during the RL stage, but throughout the entire process, including the pre-training stage, they still accumulated significant amounts of data.
9. Have Top AI Labs “Wasted” Too Much Computing Power?
For reasoning model / RL path, DeepSeek is the follower and OpenAI is the explorer. It's unfair to question OpenAI’s high R&D spending just because DeepSeek has lower costs. AI innovation works like a step function—followers spend much less on computing resources than explorers. DeepSeek has mentioned that its $5 million in costs is only for training, not research and experimentation.
As the pioneer, OpenAI has paved the way for the entire industry, and its experimental costs far surpass those of followers. We estimate that OpenAI spends between $500 million and $1 billion per year on compute costs for various experiments. This means OpenAI has shouldered much of the R&D and experimental expenses for the whole AI ecosystem—including startups like DeepSeek.
Source: DeepSeek-V3 Technical Report
10. How Will Top Labs Benefit from DeepSeek?
The industry’s understanding is converging, with Meta, as a latecomer in reasoning models, benefiting the most by leveraging more compute resources to catch up quickly.
OpenAI, Anthropic, and Google will likely realign their focus in response to increasing competition—improving both the efficiency of reasoning model training and inference while accelerating agent research. Although agents are much harder to advance than reasoning models, better reasoning capabilities and reliability will significantly aid their development.
Apple will also benefit from DeepSeek’s impact. With weaker AI capabilities than its competitors, Apple could directly adopt DeepSeek’s open-source small models for on-device use or use them to enhance its own edge AI models.
11. Why Should We Stay Optimistic About Compute Consumption?
The demand for intelligence is still massively underestimated—just think of challenges like cancer treatment or SpaceX’s heat shielding.
At a fundamental level, AI—whether it’s about developing intelligence or applying intelligence—is inherently compute-intensive. This isn’t something you can just optimize away; it’s a physical law of progress.
If we assume AI research talent and knowledge are evenly distributed, then whoever has more compute wins—it’s that simple.This explains why Musk is all-in on cluster expansion with xAI. Amazon just announced a major AI compute push—compute wars are the real game.
Talent drives algorithmic innovation, and open-source competition fuels greater compute investment. Following DeepSeek’s move, OpenAI—initially planning to hold off on new releases—quickly rolled out o3 Mini, with plans for the full o3 model and even potential open-sourcing. Anthropic and Google are also ramping up their RL research.DeepSeek has accelerated the industry’s shift toward new paradigms, lowering the barrier for smaller teams to experiment with RL across different domains. All the explorations need compute.
12. What Are DeepSeek’s Key Innovations?
DeepSeek R1 has made several counter-intuitive discoveries:
- Proving RL's Effectiveness: R1-Zero achieved long-horizon CoT without SFT, learning enhanced reasoning through RL alone. This marks an “aha moment” in model reasoning.
- Success without MCTs and PRM:process rewards are susceptible to reward hacking—where models achieve high rewards without meaningful learning. The key insight is that process supervision is inherently limited by human intuition, while outcome-based supervision better reflects a model's true potential.
- SFT serves as a support mechanism, R1 demonstrates that reasoning capabilities can emerge without it. R1 employs two data types:1)Cold-start data providing optimal initialization for better exploration (as RL optimizes to stay close to the original policy),2)Domain-specific synthetic data generated by R1 itself.
13. Why is DeepSeek’s Product a Game-Changer?
Both internet access and transparent Chain-of-Thought (CoT) significantly enhance user experience on their own. DeepSeek played both cards at once—a power move that set it apart from every other chatbot in the consumer space.
CoT transparency, in particular, is a major differentiator. By exposing the model’s reasoning process, it builds trust with users and makes AI more approachable, helping it break into mainstream adoption.
That said, Perplexity should have been heavily impacted by this shift, but DeepSeek’s server instability gave them an unexpected advantage. The Perplexity team reacted quickly, rolling out R-1 integration, and ended up capturing a significant portion of the overflow demand from DeepSeek R-1.
14. Do We Still Need Scale.ai for LLMs?
In R1, the amount of data used in SFT is relatively small. Using too much SFT data can actually have negative effects, but high-quality human-annotated data is still key. In the cold start phase, there were around a thousand training samples, which we believe are human-annotated. Even when data is not directly annotated by humans, expert involvement remains crucial - for instance, in designing specialized tasks for model annotation.
Reasoning models haven’t reached the true self-play stage yet, and synthetic data that can generate new intelligence is still a work in progress. DeepSeek understands the importance of data annotation and puts significant focus on it.
Sonnet 3.5 is not yet a reasoning model, but it remains the current SOTA and the best code model—and it’s all thanks to solid pre-training data.
DeepSeek’s success is also rooted in the strength of its data—when building their Deepseek-VL, they invested heavily in accumulating high-quality image-text pairs. For example, math datasets were self-collected, including 35.5M math webpages and 120B tokens. We believe that the high-quality token preparation for V3, with 14.8T tokens, follows a similar solid foundation.
15. Will Industry-Standard CUDA Be Bypassed After DeepSeek’s Breakthrough?
The limitation of GPUs drove DeepSeek to develop incredible cluster management capabilities. Their clusters don’t rely on NVLink, and the GRPO algorithm in R1 is not particularly GPU-friendly, which further supports the notion that they built their computational resources from scratch.
While DeepSeek’s hardware optimization is world-class, that doesn't mean the barriers for NVDA clusters are suddenly disappearing or that CUDA is on the way out. Most teams simply don’t have the level of capability DeepSeek possesses. The real reason NVDA's narrative is weakening is due to cost control and the timing of ASICs maturing.
16. Is OpenAI Still the Industry Leader?
Currently, yes, but its leadership in the o-series will be limited until agents make greater breakthroughs. The O-series likely differs from R1 in its reasoning process, as its inference time scaling has a search attribute.
o3 is divided into two tiers: o3-low and o3-high, referring to low and high inference time compute. The key hyperparameter here is sample size, which controls the number of attempts made during inference. Increasing this parameter boosts performance but also increases costs and reduces efficiency.
That said, this doesn’t create a fundamental lead. OpenAI needs a bigger breakthrough, which is harder to achieve as their organization grows more complex.
17. Where Will the Next Big AI Breakthrough Come From?
The next-generation models from top-tier players are crucial, but we’re already pushing the limits of Transformers. It’s unclear whether OpenAI, Anthropic, or Google can deliver a true generational leap. Even if they release models 30–50% better, it might not be enough to shift the landscape—especially when they have 10–30x more resources to begin with.
At the same time, Agent deployment is a critical battleground. Agents require long horizon, multi-step reasoning, where even a 5–10% improvement in model quality can translate into a massive real-world advantage. This is why OpenAI, Anthropic, and Google need to double down on:
- Full-stack integration of models + Agent products—think Windows + Office, where the ecosystem matters as much as the model.
- Showcasing their next-gen models, like o3 full version, Sonnet 4/3.5, and Opus, to reinforce leadership.
With so much uncertainty in AI’s next paradigm shift, top-tier AI researchers are the most valuable asset. Any organization serious about AGI must bet aggressively on what comes after Transformers. Now that pre training has been largely commoditized, breakthroughs will come from top talent + massive resources, pushing toward the next emergence of intelligence—the real Aha moment.
18. Will DeepSeek Follow OpenAI’s Path from Open-Source to Closed-Source?
Liang has made it clear: DeepSeek is committed to staying open-source. Their goal isn’t to lock AI behind closed doors; it’s about contributing to the growth of AI. "We will not close-source. We believe building a strong ecosystem is far more important," Liang says. He adds, "In the face of disruptive technologies, the moat that closed-source creates is temporary. Even if OpenAI closes its source, it won’t stop others from surpassing them."
For DeepSeek, the real value lies in the team—the knowledge, growth, and culture they create together. That’s their true moat. Liang believes open-sourcing and publishing papers don’t take anything away; for young researchers, being followed is an achievement. Open sourcing is more than just a business move—it’s a cultural one. It’s about giving back, earning respect, and attracting talent. DeepSeek’s open-source approach hasn’t hurt its products either—its consumer product hit 30 million DAUs in less than one month.
19. What Does DeepSeek’s Team and Organizational Structure Look Like?
DeepSeek’s team of 100 researchers is unique—none of them have experience working in the US , and very few come from big tech companies. Most are young, just out of their PhDs or with 2-3 years of work experience. This approach to hiring and nurturing talent is very similar to early OpenAI.
Liang has said publicly that DeepSeek looks for passion and curiosity above all else. “Many of our people’s desire to do research far exceeds their concern for money,” he said.
Innovation at DeepSeek is almost entirely bottom-up. For instance, the MLA innovation came from a young researcher’s initiative. Anyone with a valuable idea can freely access the resources they need to bring it to life.
Liang Wenfeng, is deeply technical—he writes code himself, annotates data, and even submitted the R1 paper personally. A former employee feels that everyone in the company is a fan of Liang, united by his vision and driven by a shared pursuit of AGI.
20. Last But Not Least: Vision Is More Important Than Technology
We want to emphasize that technology alone is not the defining factor, nor does it create an impenetrable moat. The true differentiator between AI models from various labs lies in their vision for the future. Vision, ultimately, is more powerful than technology.
When those who have the foresight to see what lies ahead leave, and when the leaders in the field become increasingly entangled in politics, product competition, or the challenges of scaling, smaller, more agile teams will find their moment to shine. It’s still too early to determine who will prevail and who will falter. The disruption DeepSeek has already introduced is a clear signal of this truth—AI innovation is far from finished, and no one’s position is beyond challenge.
More Blogs
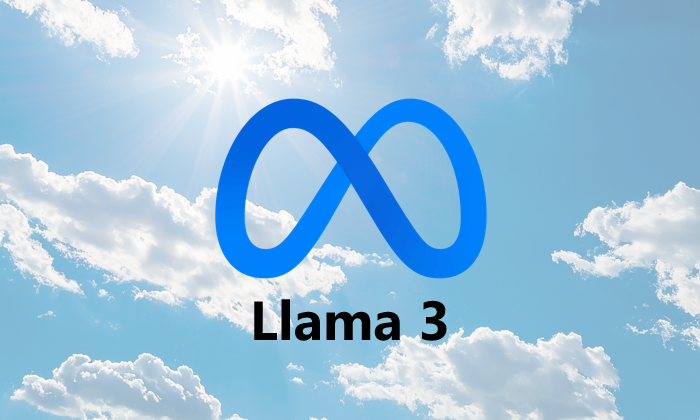
Meta's 3rd version of their open language model, Llama 3 has proven to be incredibly strong as it is even competitive with some frontier models. In this blog post, we dive into some of the technical details behind Llama 3. Additionally, we provide an in-depth overview of how the open language model compares to an array of other models, while unpacking the rationale of its immense dataset size used for training and the implications this is likely to have on scaling.
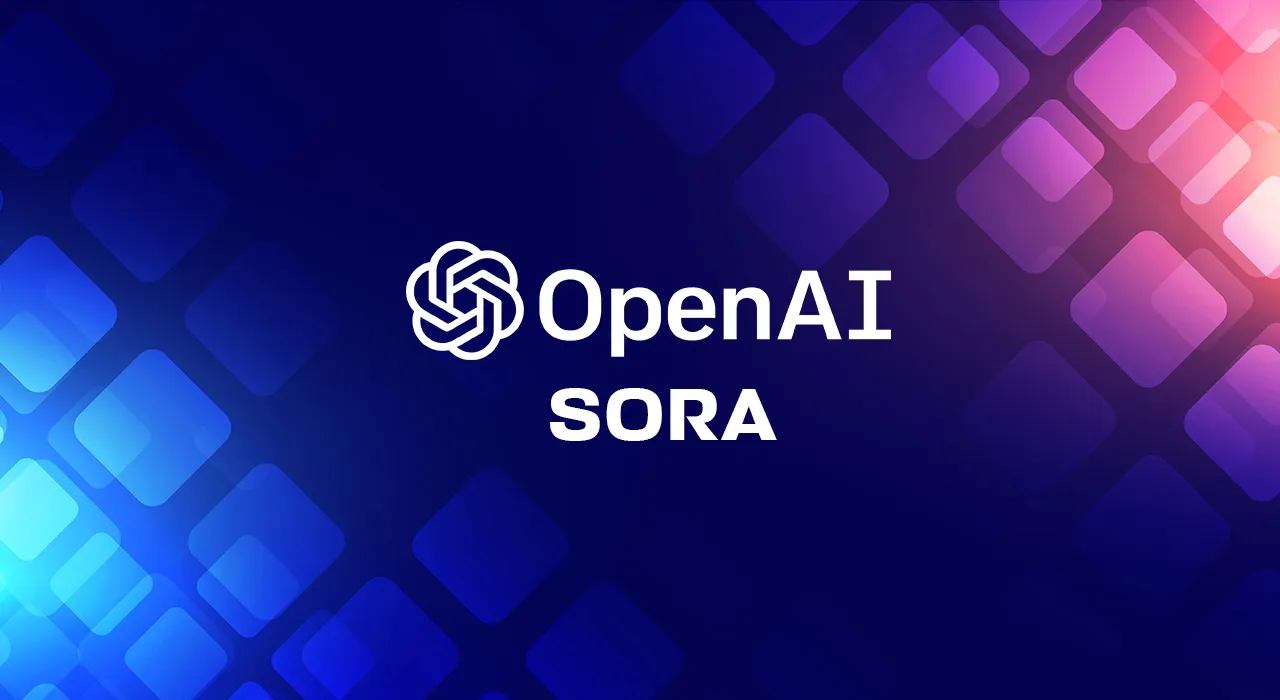
OpenAI’s Sora model has amazed the world by its ability to generate extremely realistic videos of a wide variety of scenes. In this blog post, we dive into some of the technical details behind Sora. We also talk about our current thinking around the implications of these video models. Finally, we discuss our thoughts around the compute used for training models like Sora and present projections for how that training compute compares to inference, which has meaningful indications for estimated future GPU demand.